Introduction
Artificial Intelligence (AI) is a powerful technology that has brought significant changes to our lives, especially in consumer products. However, its importance goes beyond that, as AI has also transformed industries, making them more efficient and environmentally friendly.
The real strength of AI lies in its ability to analyze huge amounts of data and find ways to improve various industrial processes. By using AI, industries have been able to make their equipment last longer, reduce operating costs, and use less energy and resources. As a result, harmful emissions have gone down, leading to a smaller impact on the environment and increased profits for companies.
In this article, we will explore the concept of “Green Intelligence (Green AI),” which shows how AI is helping us move toward a cleaner and more sustainable future.
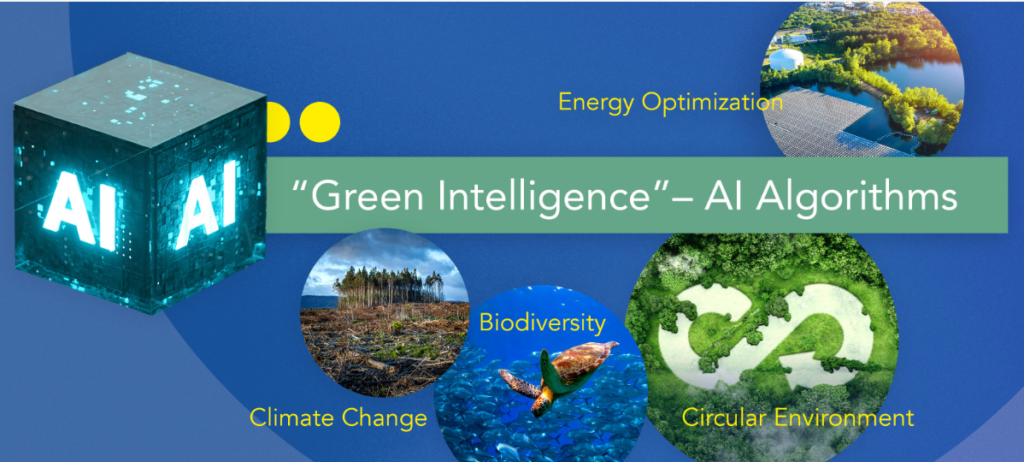
Green AI: The Emergence of Green Intelligence
The term “Green AI” refers to creating and using artificial intelligence (AI) in an eco-friendly and sustainable way. Its goal is to lessen the environmental impact of AI models and systems by reducing carbon emissions and energy usage.
Key Points in Green AI
01. Reducing computational cost:
Green AI places a strong emphasis on developing artificial intelligence (AI) models and algorithms that require less computational resources. This is vital because traditional AI models can be quite resource-intensive, consuming substantial amounts of computing power and energy.
To address this challenge, Green AI focuses on various strategies to enhance efficiency. One approach involves optimizing algorithms, making them smarter and more streamlined, so they can accomplish tasks using fewer computations. This not only speeds up the AI’s decision-making process but also reduces the overall computational burden.
Furthermore, Green AI seeks to minimize the time it takes to train AI models. Training AI models is a resource-intensive process, often involving massive datasets and complex computations. By finding ways to shorten the training time without compromising accuracy, Green AI aims to lessen the strain on computing resources.
Another critical aspect of Green AI is reducing energy consumption during inference. Inference refers to the AI’s ability to make predictions and decisions based on its learned knowledge. By devising energy-efficient inference mechanisms, AI systems can operate with minimal power consumption, contributing to a more sustainable approach.
02. Environmental sustainability
Environmental sustainability lies at the core of Green AI, with a focus on reducing the carbon footprint and environmental impact of AI systems.
To achieve this, Green AI adopts a two-pronged approach. Firstly, it advocates for the use of renewable energy sources during the training and inference processes of AI models. Instead of relying solely on energy derived from fossil fuels, which contribute to greenhouse gas emissions, Green AI promotes harnessing clean and renewable energy, such as solar, wind, or hydroelectric power. By transitioning to eco-friendly energy sources, AI operations can significantly decrease their carbon footprint and lessen their negative impact on the environment.
Secondly, Green AI endeavors to develop and utilize energy-efficient hardware for AI systems. Traditional hardware used in computing centers and data centers can consume substantial amounts of electricity. However, through advancements in hardware design and manufacturing, AI researchers and developers can create more energy-efficient processors, GPUs, and other components. These energy-efficient hardware solutions consume less power during AI computations, contributing to a more sustainable technology landscape.
By combining renewable energy sources and energy-efficient hardware, Green AI not only works towards minimizing the environmental impact of AI systems but also sets the path towards a greener and more sustainable future. Emphasizing environmental sustainability in AI development is crucial to ensure that technological advancements go hand in hand with responsible ecological stewardship.
03. Hard-coding abstract reasoning:
Green AI explores the possibility of hard-coding abstract reasoning capabilities, emulating the way human brains think, which can lead to decreased data storage requirements in data centers.
In traditional AI systems, vast amounts of data are typically required to train and fine-tune models to perform specific tasks accurately. Storing and processing such large datasets in data centers can be resource-intensive and contribute to higher energy consumption.
To address this challenge, Green AI investigates the potential of hard-coding abstract reasoning into AI algorithms. Instead of relying solely on data-driven learning, this approach involves incorporating human-like reasoning abilities directly into the AI model. By doing so, AI systems can rely less on extensive datasets for every task and perform more abstract and generalized thinking, similar to how human brains deduce and reason about various situations.
Hard-coding abstract reasoning can significantly reduce the need for storing massive datasets, consequently decreasing the data center’s storage requirements. This, in turn, leads to reduced energy consumption and a smaller carbon footprint associated with data management in AI operations.
While hard-coding abstract reasoning is a promising avenue for creating more efficient AI systems, it remains an ongoing area of research and development within the Green AI paradigm. As AI continues to evolve, integrating aspects of human-like reasoning could contribute to more sustainable and environmentally conscious AI practices.
04. Incorporating uncertainty
Green AI acknowledges the unpredictable nature of the real world and emphasizes the incorporation of uncertainty into AI systems to effectively handle complex and uncertain real-world data.
In the real world, data can be messy and unpredictable, making it challenging for traditional AI systems to cope with every possible scenario accurately. Green AI seeks to address this issue by introducing built-in uncertainty into AI models and algorithms. By considering uncertainty as a fundamental aspect, AI systems become more adaptable and robust when confronted with diverse and unpredictable data.
Incorporating uncertainty enables AI to make more nuanced decisions, recognizing that not all situations can be precisely predicted or categorized. This approach empowers AI models to provide more realistic and context-aware outcomes, even in ambiguous or unfamiliar situations.
By embracing uncertainty, Green AI strives to improve the reliability and generalizability of AI systems, making them better suited for real-world applications. This ability to handle uncertainty effectively reduces the risk of AI models making inaccurate or biased predictions, contributing to safer and more trustworthy AI technologies. As a result, Green AI fosters the development of AI systems that can navigate the intricacies of the real world in an environmentally responsible manner.
05. Promoting inclusivity
Green AI promotes inclusivity by advocating for AI research and development that is accessible to a broader spectrum of individuals, including those with limited computational resources. It strives to foster a shift towards environmentally friendly AI practices.
In traditional AI research, access to cutting-edge technology and computational resources can be a significant barrier for many researchers and developers, particularly those from less privileged backgrounds or regions with limited access to advanced hardware. Green AI recognizes this disparity and seeks to level the playing field by encouraging the adoption of AI practices that require fewer resources.
By promoting inclusivity, Green AI aims to democratize AI research and development, allowing more individuals to contribute to the advancement of AI technologies. This broader participation can lead to a wider variety of perspectives and innovative ideas, enriching the AI community as a whole.
Furthermore, embracing environmentally friendly AI practices ensures that the research and development process is mindful of its ecological impact. By optimizing algorithms, using energy-efficient hardware, and considering the environmental consequences of AI solutions, researchers can work towards creating AI technologies that are not only accessible but also sustainable.
Ultimately, Key Point 5 emphasizes the importance of making AI more inclusive and environmentally conscious. By opening up AI research to a diverse range of individuals and considering the environmental implications of AI development, Green AI aims to build a more equitable and ecologically responsible future for artificial intelligence.
Summary
In conclusion, Green AI aims to create AI systems that are both powerful and eco-friendly, taking into account the planet’s resources. This involves optimizing algorithms to be more efficient, reducing energy usage, and prioritizing sustainability in AI research and development. By embracing Green AI principles, we can build a more sustainable and responsible future for artificial intelligence, benefiting both our technological advancements and the well-being of the environment.